Synthetic Data in Medical Imaging
- Sadegh Mohammadi
- Dec 6, 2024
- 11 min read
Updated: Jan 13
For a quick overview, check out the inforgraphic, that summarizes the key points of the post.
The transformative potential of AI in healthcare is immense, especially in medical imaging. I believe technology holds the key to making healthcare more accessible and equitable. Today, I’d like to explore how we can address one of the most persistent challenges—data scarcity—and move closer to a future where medical imaging is universally accessible and unrestricted.
Imagine the World, Where Medical Imaging isn't just Widespread....
At that time, doctors relied solely on physical exams, patient descriptions, and limited tools to diagnose hidden conditions. Diagnosing internal issues was a daunting task, often requiring invasive surgery to see what was happening beneath the surface. Everything changed with the discovery of X-rays by Wilhelm Röntgen in 1895, allowing doctors a non-invasive glimpse inside the body.
Today, imaging technologies like CT scans and MRIs have transformed healthcare, enabling early diagnosis, guiding surgeries, and improving patient outcomes. The leap from guesswork to precise visualization underscores the profound impact of medical imaging on modern medicine.
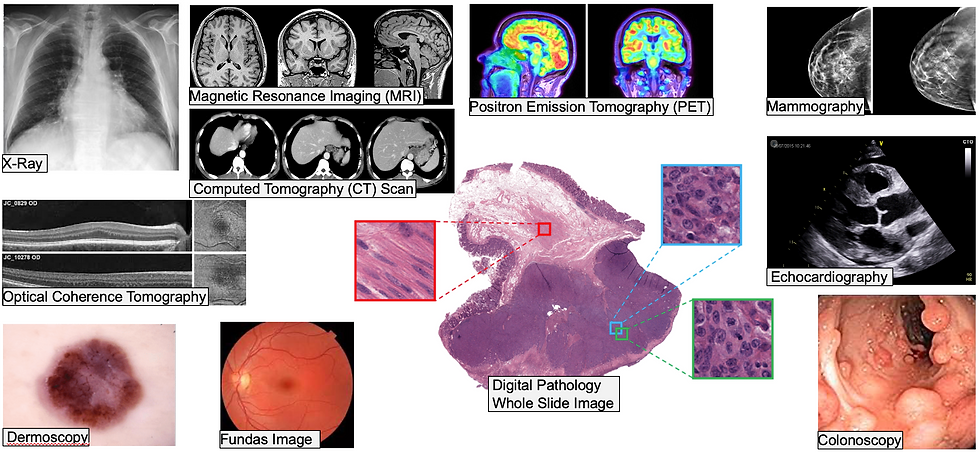
The Role of AI in Shaping the Future of Medical Imaging
Despite significant advancements in imaging technologies, radiologists are under increasing pressure due to imaging studies' sheer volume and complexity. This is largely driven by an aging population and the rise of chronic and complex diseases. As a result, delays, stress, and burnout are becoming alarmingly common. In fact, in 2023, nearly 54% of radiologists reported burnout, up from 49% the previous year [1].
AI offers a transformative solution by analyzing vast datasets swiftly, detecting subtle anomalies, and automating routine tasks, AI reduces radiologists' burdens, enabling them to focus on challenging cases. This not only improves diagnostic accuracy but also addresses burnout. For instance, AI-supported cancer screening has been linked to a 44% reduction in radiologists' workload without compromising detection rates [2].
The healthcare sector is increasingly recognizing these benefits. The global AI in medical imaging market, valued at USD 1.01 billion in 2023, is projected to grow at a compound annual growth rate (CAGR) of 34.8%, reaching an estimated USD 8.18 billion by 2030 [3]. However, the rapid growth of AI in medical imaging hinges on a critical prerequisite: access to high-quality data.
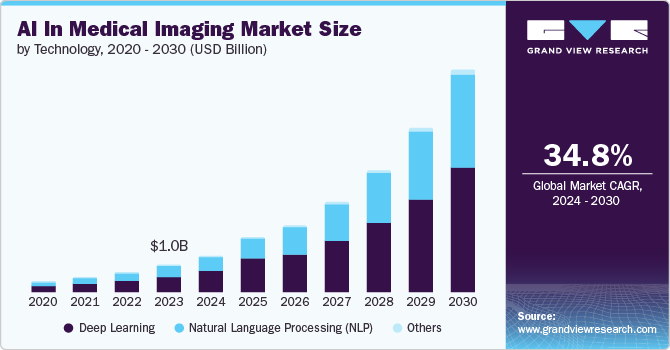
Data Scarcity: The Key Barrier and Market Opportunity for AI in Medical Imaging
While the promise of AI is undeniable, its success in medical imaging is largely constrained by data scarcity. Unlike consumer applications of AI, such as ChatGPT, medical imaging faces unique challenges related to data accessibility. Strict patient privacy regulations, the fragmented nature of imaging data across healthcare systems, and variability in imaging protocols restrict access to the large, high-quality datasets required to train robust AI models for tasks like anomaly detection, segmentation, and classification. These factors collectively impede the development of robust AI models, slowing the sector’s progress toward transformative innovation, or failure of AI initiatives in this sector [4,5].
Consider one of our major projects aimed at developing an AI algorithm to assist radiologists in analyzing contrast-enhanced MRI scans. Despite an investment of over €1.5 million and the acquisition of MRI data from 7,000 patients, only 2,000 datasets passed rigorous quality checks. The remaining 80% of the data was discarded due to issues such as heterogeneity, imbalance, and low quality. Vendor delays and logistical challenges compounded the problem, resulting in over a year’s delay. Ultimately, the project was halted—not because the AI algorithms failed, but because the value proposition became unviable, and we missed the market opportunity. We needed a smarter way to close the gap before making a significant investment...

Generative AI disrupts the Computer Vision Domain
Recent advancements in generative AI for image creation have transformed various fields, including art, fashion, and film, by enabling the production of highly realistic visuals from simple text prompts. For instance, Adobe has introduced AI tools within its stock photography business, allowing customers to create and modify images using its stock library while compensating the original creators [6]. In the film industry, AI is used to de-age actors, as seen in the movie "Here", where generative AI was employed to make Tom Hanks look younger [7].
Now, you might argue that while Generative AI excels at creating realistic-looking images and seamlessly editing visuals, it may not yet be capable of producing reliable medical imaging. To address this concern, we conducted comprehensive research, which I’ll share throughout this blog. Before diving into the use case, it’s important to first introduce the core technology behind our work: Diffusion Models.

Diffusion Models: The Secret Behind AI's Imaging Breakthroughs
Diffusion models are a breakthrough in AI image generation. A notable example is OpenAI's DALL·E series, Google's Imagen, and Stability ai's Stable Diffusion Models which utilize diffusion models to produce detailed images based on textual prompts. Diffusion Models are built on three key components: forward diffusion, reverse diffusion, and texture embedding. In forward diffusion, noise is progressively added to an image, transforming it into a completely degraded version. Reverse diffusion then reconstructs the image by iteratively removing noise, starting from a fully noised state and refining it step by step until the image is clear, akin to an artist adding details to a blank canvas. The standout feature of diffusion models is their ability to integrate text prompts, enabling users to guide image creation with descriptive inputs. By training on millions of images, these models learn to generate highly realistic visuals based on user prompts, similar to how ChatGPT generates images based on the text prompt.

Our first attempt: Histopathology Meets Innovation
Our initial experiments began with the off-the-shelf Stable Diffusion model, originally trained on datasets featuring artistic imagery. When we applied it to generate synthetic histopathology images, we observed a fascinating phenomenon: the model produced artistic-looking representations of histopathology. While these images were not suitable for medical use, they revealed an unexpected capability of diffusion models. Despite lacking any prior exposure to medical imaging, the model demonstrated a creative attempt to 'think' beyond its training, striving to generate synthetic histopathology images. This experiment highlights the model's remarkable adaptability and the potential to refine such generative AI systems for domain-specific applications. Having said that as a next step, we decided to refine the existing model of histopathology
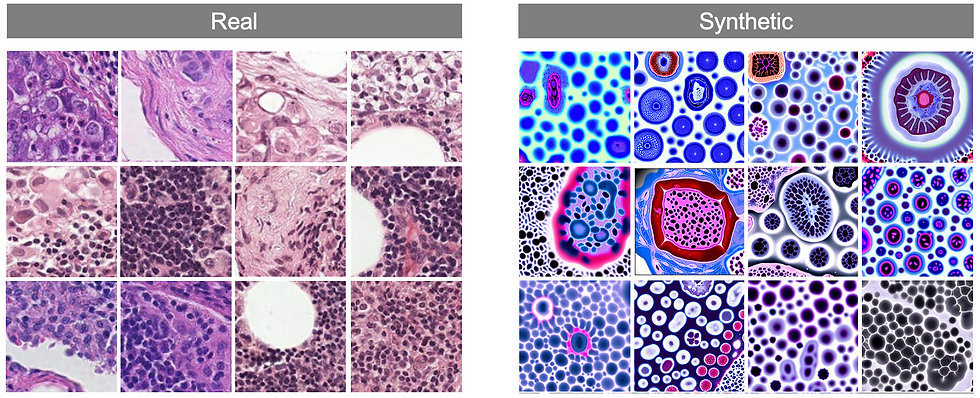
Training Diffusion Model on the Histopathology Images
As the next step, we set out to adapt the existing diffusion model for histopathology-specific datasets. Given the sheer size of histopathology images, processing them directly was beyond the capabilities of our available computational resources. To address this, we extracted smaller patches from the original images and labeled them as either healthy or cancerous tissue. To align with the requirements of diffusion models, we paired each image patch with descriptive texture prompts such as 'Histopathology Healthy' or 'Histopathology Cancer Type 1...10.'
With this setup, we fed the labeled patches and their corresponding prompts into the model, applying the forward and reverse processes just as we would for generating natural images. This approach allowed the model to begin learning the unique visual characteristics of histopathology, setting the foundation for generating meaningful synthetic medical images tailored to this domain. If you are interested in the methodology behind this work please review our latest work [8]

Resounding Success: Qualitative Triumphs on the Human Benchmark
To assess the quality of the generated images, we conduct a visual turning study. A Visual Turing Test involving five board-certified veterinary pathologists assessed the plausibility of synthetic images generated through the Diffusion models. Presented with 20 real and 20 synthetic images, pathologists classified each image as “definitely real,” “maybe real,” “maybe synthetic,” or “definitely synthetic” without prior exposure or additional context. The experiment revealed no significant ability to differentiate real from synthetic images, demonstrating the high quality and plausibility of the synthetic dataset.
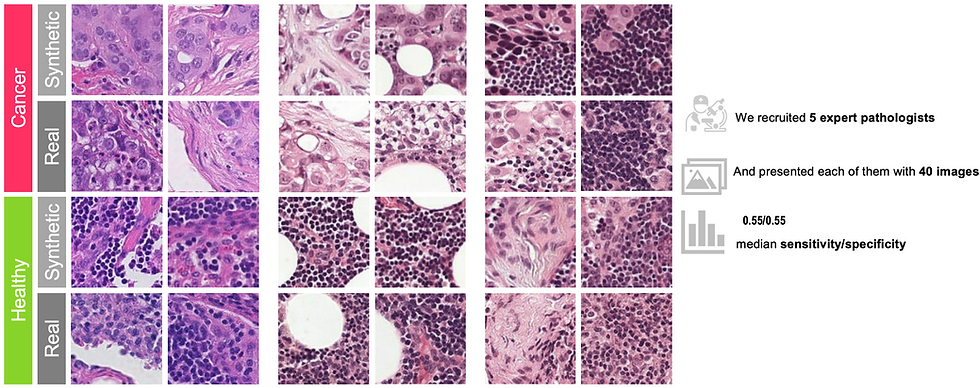
I strongly encourage readers to explore our detailed work titled: "Latent Diffusion Models with Image-Derived Annotations for Enhanced AI-Assisted Cancer Diagnosis in Histopathology" for a comprehensive understanding of this research.
Beyond histopathology, our research extends to include comprehensive studies on Chest X-rays. While the specifics are outside the scope of this blog, you can explore the details in our publication titled: DiNO-Diffusion. Scaling Medical Diffusion via Self-Supervised Pre-Training
Looking Forward: Opportunities and Risks
I firmly believe that from a technological perspective, Generative AI has reached a level of maturity where it can produce highly realistic synthetic images. However, when it comes to medical imaging for applications that directly impact healthcare decisions, the sensitivity of regulatory barriers presents a significant challenge. That said, waiting for the regulatory landscape to fully develop before integrating this technology into workflows would be a missed opportunity. With such a disruptive innovation, regulatory frameworks are evolving rapidly, and agencies are increasingly open to considering innovative approaches. Now, let’s delve into a detailed discussion of the opportunities and risks involved.
Opportunities:
There are three immediate use cases and one future-looking use case that I am currently pushing for immediate use cases that could directly leverage from Synthetic Medical Imaging.
1) Guided Data Acquisition Investment: Acquiring real-world data is costly and time-intensive. We can adopt an incremental approach instead of immediately engaging multiple data brokers. This involves leveraging a small dataset acquired externally or from in-house sources to conduct a proof-of-concept. Generative AI can then be used to create synthetic images, allowing us to assess the impact of additional data. You might argue that Synthetic data is generated via limited available real images and does not bring significant value. I agree with the statement, however, despite data scarcity, adding additional data still must provide a positive signal of improvement. If the results show a positive signal, we can proceed with further investment and data acquisition, ensuring resources are used efficiently and effectively.

2) Educational Purpose: Studying human bodies is complex and radiologists need significant training to build expertise around various diseases. However, many diseases are rare and they do not have to be trained in various conditions. Therefore, creating Synthetic Medical Images that create Synthetic diseases with various lesions can be a way forward to train radiologists in rare complex cases.
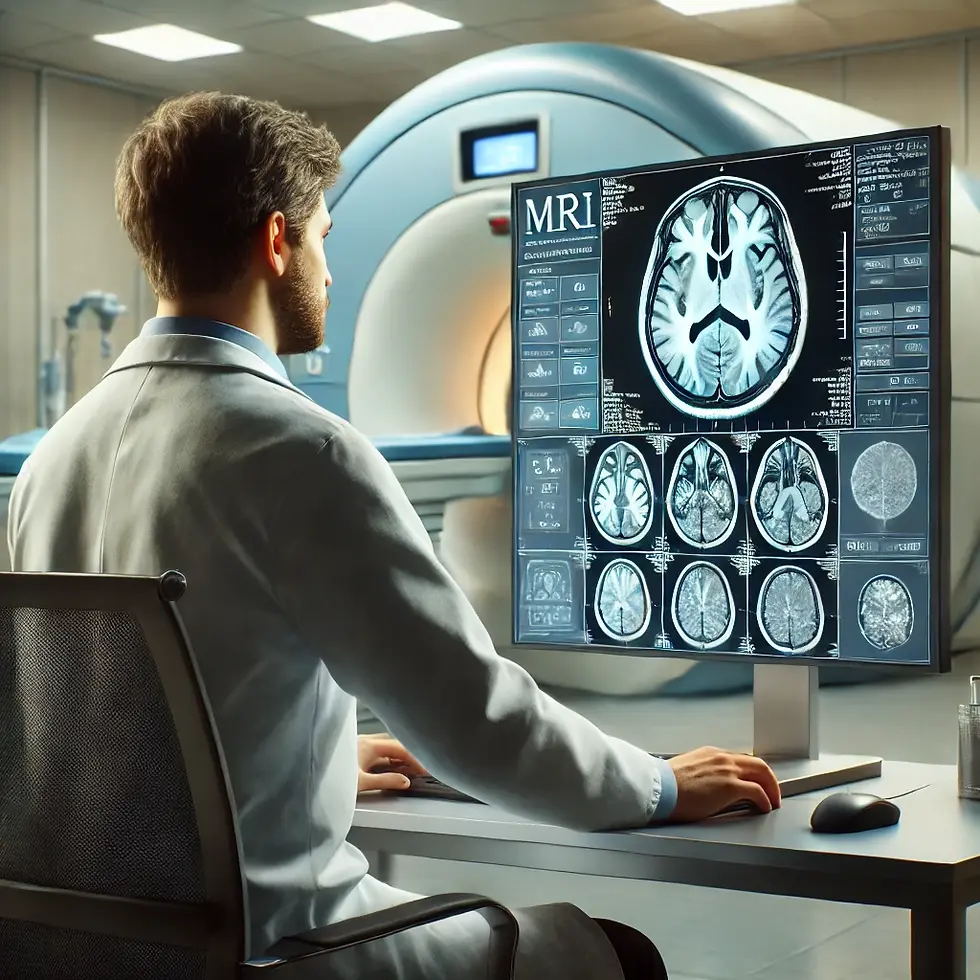
3) Accelerating external collaboration and data sharing: In my experience collaborating with third parties is challenging and time-consuming, and I have been witness to delaying various AI initiatives when it comes to external collaborators, mainly due to the data sharing barriers even after having a legal contract in place. To accelerate this process we could generate anonymized Synthetic Images based on the real data to share with the third parties to kick off the project as soon as possible while we are dealing with our internal process to make the real data available to the collaborator. Moreover, we could even move beyond our collaborator and provide the Synthetic Images as an open-source tool that can be used for research purposes.
Risks:
Despite the significant excitement around Generative AI and its capability in Generating Synthetic Images, some major concerns have been reported in the research community that is essential to be taken under consideration. Below I have listed three major challenges in which the first two- Model Collapse and Haullicination are considered as technical challenges while Deepfake is an important ethical consideration that we must take into account.
Model Collapse [10]: refers to the phenomenon where the generative model produces outputs that lack diversity, often converging to a limited set of patterns or even a single mode. This results in generated samples that are repetitive and fail to capture the full variability of the target distribution. We observed model collapse in the Digital Pathology project, that model feels comfortable with one specific pattern within the training data and it keeps generating the same images regardless of the user instruction in generating images via prompt.
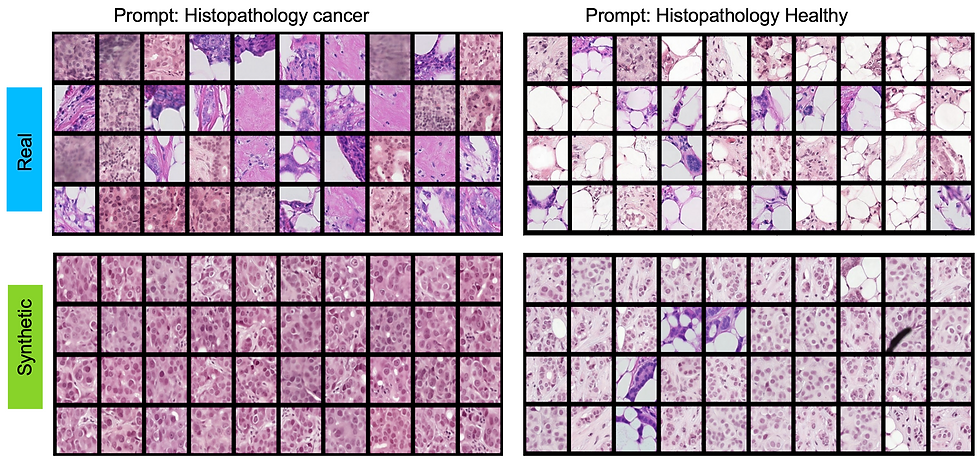
Model Hallucination [9]: refers to instances where Generative AI systems produce outputs that are false, misleading, or not grounded in their training data. This phenomenon occurs when the model generates information that appears plausible but lacks factual accuracy or relevance. In the context of medical imaging models can generate synthetic images or features that do not accurately represent the underlying biological structures, potentially leading to misinterpretations and diagnostic errors.
Deepfakes: refer to a synthetic media—such as an image, video, or audio recording—created using artificial intelligence techniques, particularly deep learning, to convincingly mimic real individuals or events, often making it challenging to distinguish from authentic content. The advent of generative AI technologies, particularly deepfakes, in medical imaging has introduced significant ethical concerns. Recent research has demonstrated the capability to generate synthetic images that can artificially map lesions onto patients' CT scans, raising the potential for misuse in falsifying medical records. Such manipulations could lead to fraudulent insurance claims, misinform clinical trials, or deceive healthcare providers, ultimately compromising patient care and trust in medical systems.

Closing Note:
We are in an exciting time when technology such as Generative AI is developing so fast in research and industry communities. However, when it comes to healthcare, especially in medical imaging, there is a long way to go to leverage the potential of technology fully. The human body is a complex puzzle, it comprises approximately 78 organs, each vulnerable to a multitude of diseases. Medical imaging modalities—such as X-rays, CT scans, MRI, and ultrasound—are essential for diagnosing a wide range of pathologies across these organs. Each organ, imaging modality, and pathology presents unique technical challenges from a Data Science perspective. For example, conditions affecting larger, more anatomically distinct organs like the lungs may produce datasets that are easier to standardize and replicate. In contrast, rare diseases or those involving subtle, microscopic changes, such as certain cancers or genetic disorders, pose significant challenges in terms of data scarcity and variability.
On the other hand, regulatory frameworks must address technical and ethical concerns, including data privacy, potential biases, and the authenticity of synthetic images. However, Traditional validation processes often fall short in assessing the complexities of synthetic data, making it crucial to develop methods that evaluate its realism and applicability [12].
Therefore, collaboration among subject matter experts, Data Scientists, and regulatory bodies is the key to leveraging the full potential of Synthetic Medical Imaging.
Call for Action:
If you are interested in the area of Synthetic data generation do not hesitate to reach out to me via Email or through Linkedin.
Comments